As a data scientist, I’ve always been fascinated by the power of analytics to glean insights and drive impactful decisions. But it wasn’t until I stumbled upon a case study involving a major e-commerce platform that I truly understood the crucial role of causal analysis. They had implemented a new marketing campaign, and while their initial data showed a spike in sales, it turned out that the increase was largely due to seasonal fluctuations, not the campaign itself. This realization highlighted the importance of understanding the *why* behind observed changes, rather than just relying on correlations.
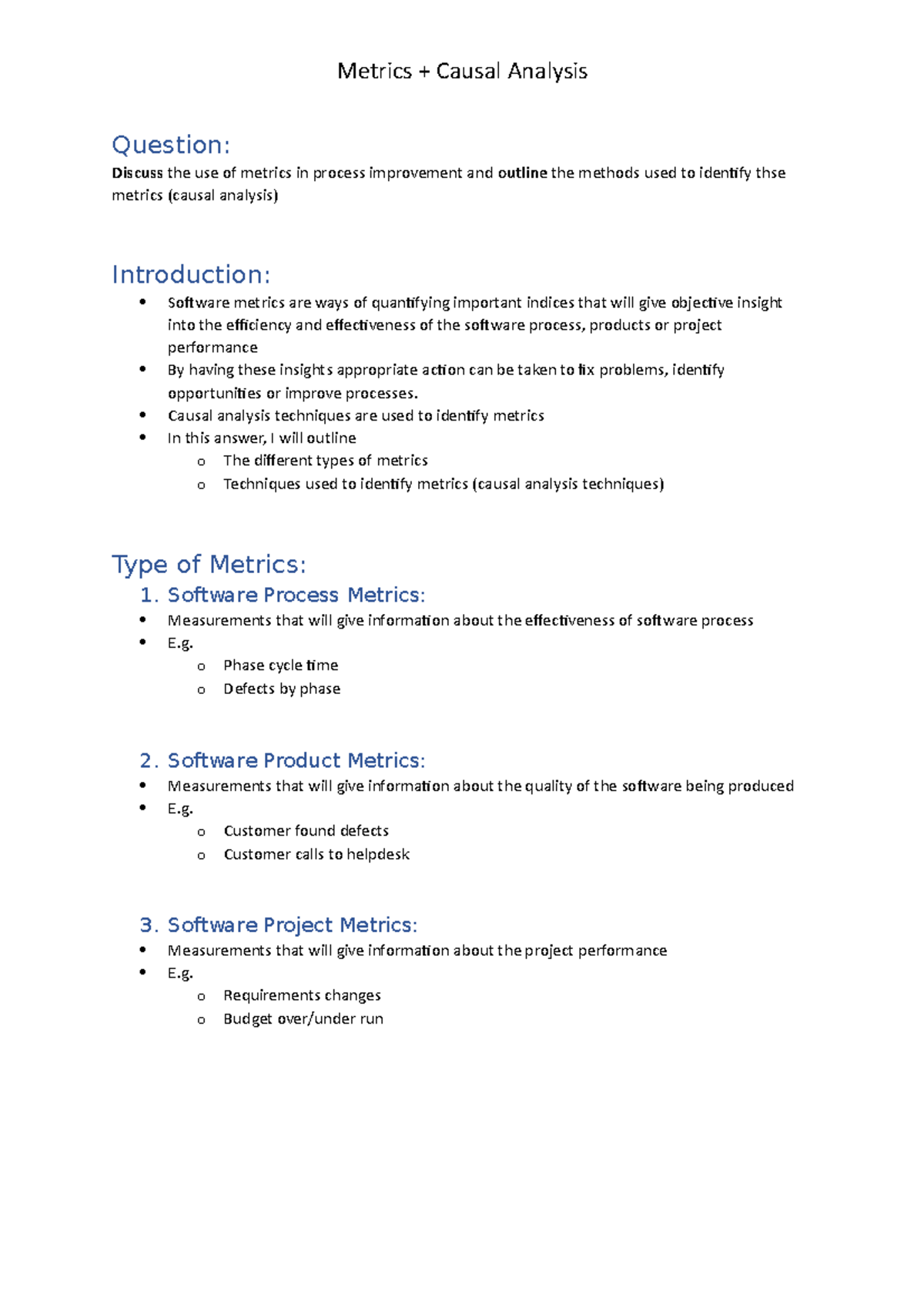
Image: www.studocu.com
This is where causal analysis comes into play, providing a framework for establishing a true cause-and-effect relationship between variables. It’s not just about identifying patterns, but about disentangling complex systems and understanding the underlying drivers of change.
The Importance of Causal Analysis in Data Interpretation
Causal analysis goes beyond mere correlation, seeking to prove a genuine cause-and-effect relationship between variables. It helps us understand not just “what” happened, but “why” it happened. This understanding is crucial for informed decision-making, especially in business, healthcare, and social sciences, where interventions and actions are often based on data analysis.
For instance, in marketing, a company might observe a rise in sales following a new ad campaign. However, this could be influenced by external factors like a seasonal surge or competitor activity. A causal analysis can help determine the true impact of the campaign by isolating its effects from other variables. This allows companies to optimize their marketing strategies for better ROI.
Understanding Causal Analysis: A Closer Look
Causal analysis is a powerful tool for understanding complex systems, and it is essential for accurate data interpretation and effective decision-making. While it might seem daunting, it can be broken down into key concepts and its application can be easily understood with various examples.
What is Causal Analysis?
Causal analysis is a scientific method used to establish a causal relationship between two or more variables. It goes beyond simply observing correlation, aiming to demonstrate that a change in one variable directly leads to a change in another, eliminating any potential confounding factors.
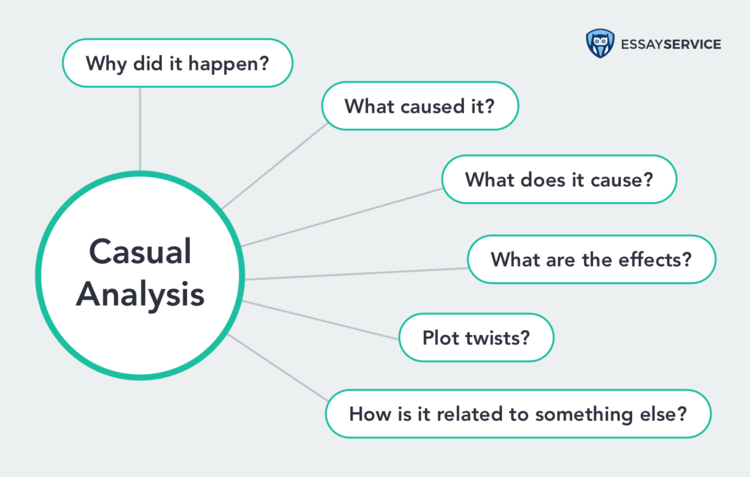
Image: jamieyouthballard.blogspot.com
Why is Causal Analysis Mandatory?
Causal analysis is crucial for making informed and effective decisions. Without a proper understanding of cause and effect, we risk drawing incorrect conclusions and implementing ineffective strategies. It helps us to understand the underlying mechanisms driving change, enabling us to predict future outcomes with greater accuracy and make informed decisions.
Techniques of Causal Analysis
Many techniques are employed in causal analysis, each with its own strengths and limitations. These include:
- Regression analysis: This statistical technique helps identify the relationship between a dependent variable and one or more independent variables, accounting for potential confounding factors.
- Natural experiments: These leverage naturally occurring events or changes as treatment groups, allowing researchers to observe the causal effects by comparing outcomes between groups.
- Randomized controlled trials (RCTs): This gold standard approach involves randomly assigning participants to treatment and control groups, minimizing bias and providing strong evidence for causality.
- Instrumental variable analysis: This method uses instrumental variables, which are correlated with the treatment but not directly related to the outcome, to isolate the causal effect of the treatment.
Causal Analysis in Action: Examples from Different Fields
Causal analysis is applied across various fields, influencing decisions and shaping our understanding of the world.
Healthcare
In healthcare, causal analysis is used to assess the effectiveness of new treatments and interventions. For instance, a study might investigate the causal relationship between a new drug and a specific disease outcome. Causal analysis is essential to identify if the drug actually benefits patients or if the observed improvement is due to other factors.
Marketing
In marketing, causal analysis helps decipher the impact of various marketing strategies on sales and brand awareness. For example, a company might conduct an experiment to assess the effectiveness of email marketing campaigns on customer engagement. Using causal analysis techniques, they can determine if the increase in engagement is directly attributable to the email campaign or influenced by other factors.
Economics
In economics, causal analysis is instrumental in evaluating the impact of economic policies on various indicators like GDP, inflation, and unemployment. For example, researchers might analyze the causal relationship between changes in interest rates and economic growth. Understanding these causal relationships helps policymakers make informed decisions about economic policies.
Trends and Developments in Causal Analysis
The field of causal analysis is constantly evolving, with new methods and applications emerging. Some key developments include:
- Machine learning methods for causal inference: Machine learning algorithms are increasingly being used to perform causal inference. These methods can handle complex data structures and identify intricate causal relationships.
- Causal mediation analysis: This approach goes beyond identifying direct causal effects, examining the pathways and mechanisms through which an intervention impacts an outcome.
- Causal Bayesian networks: These models represent causal relationships in a graphical format, allowing for a more intuitive and comprehensive understanding of the causal structure of a system.
Expert Tips and Advice: Making Causal Analysis Work for You
While causal analysis might seem complex, integrating it into your data-driven decision-making process is more approachable than you might think. Here are some tips to get started:
- Define your research question clearly: Clearly articulate the specific causal relationship you are trying to investigate to guide data collection and analysis.
- Consider potential confounding factors: Identify and account for variables that might influence the relationship between your variables of interest.
- Choose the right method: Select an appropriate causal analysis technique based on your research question, data structure, and available resources.
- Interpret results cautiously: Remember that even the strongest causal evidence may not guarantee a perfect understanding of the underlying system.
The power of causal analysis lies not just in advanced techniques, but in a clear understanding of your research question and a willingness to consider potential confounders. Remember, the goal is not to prove a predetermined outcome, but to uncover the truth behind your data.
FAQ:
What are some examples of causal analysis in everyday life?
One example is if you’re trying to understand why you feel tired after a heavy meal. Causal analysis suggests that consuming a large, high-carbohydrate meal could lead to fatigue due to the body’s response in processing the food. Identifying your specific trigger and understanding the underlying cause helps you find solutions to manage your energy levels.
What is the difference between causal analysis and correlation?
Correlation simply indicates that two variables change together, while causal analysis seeks to prove that a change in one variable directly causes a change in another. Correlation can exist without causation, but causation always implies correlation.
What are some common pitfalls to avoid in causal analysis?
One pitfall is assuming causation based solely on correlation without considering other potential explanations. Another is failing to account for confounding factors, leading to unreliable conclusions.
Causal Analysis Is Mandatory For Which Of The Below Metrics
Conclusion
Causal analysis is an essential tool for extracting actionable insights from data. It moves beyond correlation, seeking to establish a true cause-and-effect relationship. By understanding the “why” behind observed changes, we can make more informed decisions and develop more effective strategies.
Whether you’re a data scientist, marketer, or simply someone who wants to understand the world around them better, causal analysis can empower you to make smarter choices, navigate complex systems, and contribute to meaningful change.
Are you interested in learning more about causal analysis and its applications in your field? Let me know your thoughts in the comments below!